Claim Optimization in Computational Argumentation
Gabriella Skitalinskaya, Maximilian Spliethöver, Henning Wachsmuth
In Sessions:
INLG Oral Session 5: NLG for real-world applications: (Friday, 11:30 CEST, Sun II , Watch on Zoom , Chat on Discord )
Poster
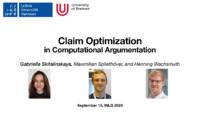
Abstract:
An optimal delivery of arguments is key to persuasion in any debate, both for humans and for AI systems. This requires the use of clear and fluent claims relevant to the given debate. Prior work has studied the automatic assessment of argument quality extensively. Yet, no approach actually improves the quality so far. To fill this gap, this paper proposes the task of claim optimization: to rewrite argumentative claims in order to optimize their delivery. As multiple types of optimization are possible, we approach this task by first generating a diverse set of candidate claims using a large language model, such as BART, taking into account contextual information. Then, the best candidate is selected using various quality metrics. In automatic and human evaluation on an English-language corpus, our quality-based candidate selection outperforms several baselines, improving 60% of all claims (worsening 16% only). Follow-up analyses reveal that, beyond copy editing, our approach often specifies claims with details, whereas it adds less evidence than humans do. Moreover, its capabilities generalize well to other domains, such as instructional texts.