GAN-LM: Generative Adversarial Network Using Language Models for Downstream Applications
Dae Yon Hwang, Yaroslav Nechaev, Cyprien de Lichy, Renxian Zhang
In Sessions:
INLG Oral Session 5: NLG for real-world applications: (Friday, 11:30 CEST, Sun II , Watch on Zoom , Chat on Discord )
Poster
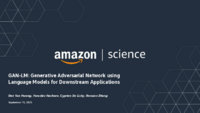
Abstract:
In this work, we investigate Data Augmentation methods to improve the performance of state-of-the-art models for four different downstream tasks. Specifically, we propose Generative Adversarial Network using Language Models (GAN-LM) approach that combines a deep generative model with a pre-trained language model to produce diverse augmentations. We compare the GAN-LM to various conventional methods in non-contextual- and contextual-levels on four public datasets: ZESHEL for zero-shot entity linking, TREC for question classification, STS-B for sentence pairs semantic textual similarity (STS), and mSTS for multilingual sentence pairs STS. Additionally, we subsample these datasets to study the impact of such augmentations in low-resource settings where limited amounts of training data is available. Compared to the state-of-the-art methods in downstream tasks, we mostly achieve the best performance using GAN-LM approach. Finally, we investigate the way of combining the GAN-LM with other augmentation methods to complement our proposed approach. The developed code for reproducibility is included in the supplementary material.