Guidance in Radiology Report Summarization: An Empirical Evaluation and Error Analysis
Jan Trienes, Paul Youssef, Jörg Schlötterer, Christin Seifert
In Sessions:
INLG Oral Session 1: Trustworthiness of NLG systems: (Wednesday, 10:45 CEST, Sun II , Watch on Zoom , Chat on Discord )
Poster
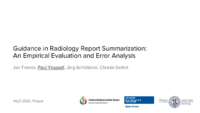
Abstract:
Automatically summarizing radiology reports into a concise impression can reduce the manual burden of clinicians and improve the consistency of reporting. Previous work aimed to enhance content selection and factuality through guided abstractive summarization. However, two key issues persist. First, current methods heavily rely on domain-specific resources to extract the guidance signal, limiting their transferability to domains and languages where those resources are unavailable. Second, while automatic metrics like ROUGE show progress, we lack a good understanding of the errors and failure modes in this task. To bridge these gaps, we first propose a domain-agnostic guidance signal in form of variable-length extractive summaries. Our empirical results on two English benchmarks demonstrate that this guidance signal improves upon unguided summarization while being competitive with domain-specific methods. Additionally, we run an expert evaluation of four systems according to a taxonomy of 11 fine-grained errors. We find that the most pressing differences between automatic summaries and those of radiologists relate to content selection including omissions (up to 52%) and additions (up to 57%). We hypothesize that latent reporting factors and corpus-level inconsistencies may limit models to reliably learn content selection from the available data, presenting promising directions for future work.