This Is Not Correct! Negation-Aware Evaluation of Language Generation Systems
Miriam Anschütz, Diego Miguel Lozano, Georg Groh
In Sessions:
INLG Oral Session 4: Evaluation and linguistic analysis of NLG systems: (Thursday, 14:00 CEST, Sun II , Watch on Zoom , Chat on Discord )
Poster
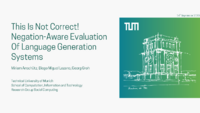
Abstract:
Large language models underestimate the impact of negations on how much they change the meaning of a sentence. Therefore, learned evaluation metrics based on these models are insensitive to negations. In this paper, we propose NegBLEURT, a negation-aware version of the BLEURT evaluation metric. For that, we designed a rule-based sentence negation tool and used it to create the CANNOT negation evaluation dataset. Based on this dataset, we fine-tuned a sentence transformer and an evaluation metric to improve their negation sensitivity. Evaluating these models on existing benchmarks shows that our fine-tuned models outperform existing metrics on the negated sentences by far while preserving their base models' performances on other perturbations.