Models of Reference Production: How Do They Withstand the Test of Time?
Fahime Same, Guanyi Chen, Kees van Deemter
In Sessions:
INLG Oral Session 4: Evaluation and linguistic analysis of NLG systems: (Thursday, 14:00 CEST, Sun II , Watch on Zoom , Chat on Discord )
Poster
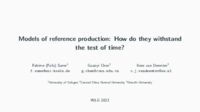
Abstract:
In recent years, many NLP studies have focused solely on performance improvement. In this work, we focus on the linguistic and scientific aspects of NLP. We use the task of generating referring expressions in context (REG-in-context) as a case study and start our analysis from GREC, a comprehensive set of shared tasks in English that addressed this topic over a decade ago. We ask what the performance of models would be if we assessed them (1) on more realistic datasets, and (2) using more advanced methods. We test the models using different evaluation metrics and feature selection experiments. We conclude that GREC can no longer be regarded as offering a reliable assessment of models' ability to mimic human reference production, because the results are highly impacted by the choice of corpus and evaluation metrics. Our results also suggest that pre-trained language models are less dependent on the choice of corpus than classic Machine Learning models, and therefore make more robust class predictions.